
First, 64 quantitative image features, including histogram features based on basic histogram characteristics, percentages of pixels above the thresholds, percentile intensities, and texture features based on gray-level co-occurrence matrices (GLCM), gray-level run-length matrices (GLRLM), and local binary patterns (LBP), were extracted from each ROI. A feature classification system was proposed for separating two types of renal masses, using histogram and texture features and machine learning classifiers. From these images, the tumors were manually segmented by an expert radiologist to define the regions of interest (ROI).
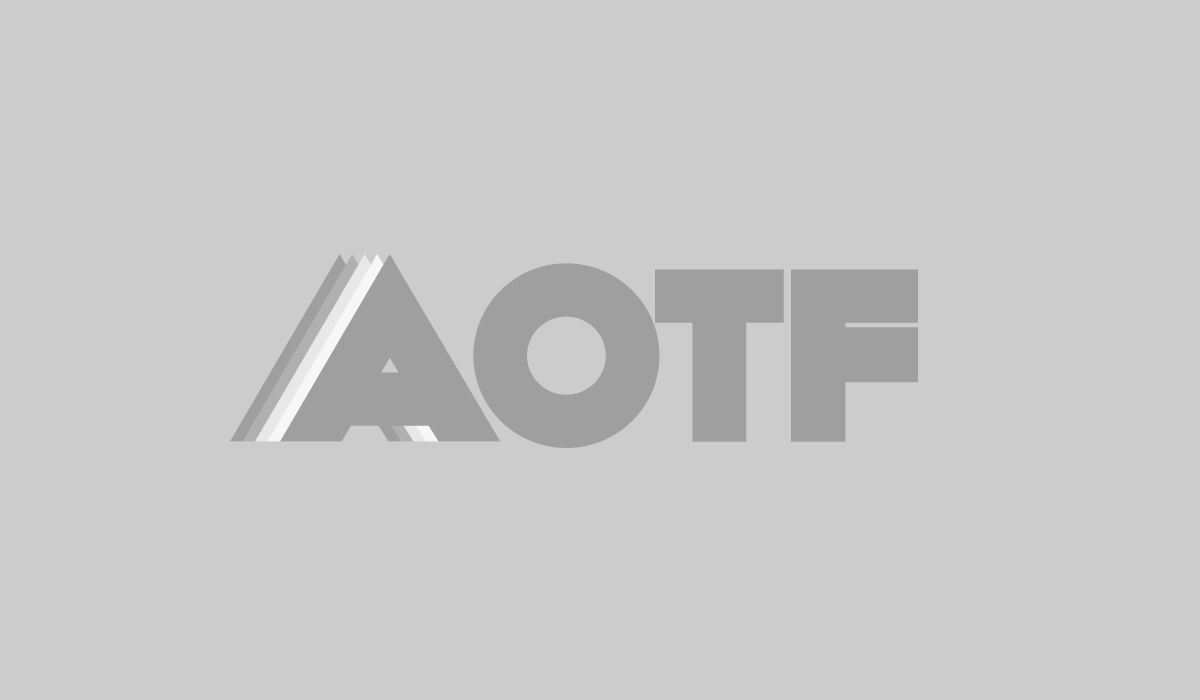
To develop a computer-aided classification system to differentiate benign fat-poor angiomyolipoma (fp-AML) from malignant clear cell renal cell carcinoma (ccRCC) using quantitative feature classification on histogram and texture patterns from contrast-enhanced multidetector computer tomography (CE MDCT) images.Ī dataset including 50 CE MDCT images of 25 fp-AML and 25 ccRCC patients was used.
